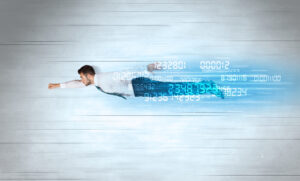
(ESB-Skilled/Shutterstock)
If information is the supply of AI, then it follows that one of the best information creates one of the best AI. However the place does one discover extremely high-quality information? In response to the oldsters at SuperAnnotate, that sort of knowledge doesn’t exist naturally. As a substitute, you could create it by enriching your present digital inventory, which is the purpose of the corporate and its product.
As its title suggests, SuperAnnotate is within the enterprise of knowledge annotation, or information labeling. That would embrace placing bounding packing containers round people in a pc imaginative and prescient use instances, or figuring out the tone of a dialog in a pure language processing (NLP) use case. However information annotation is simply only the start for SuperAnnotate, which helps automate extra information duties which are wanted to create coaching information of the best high quality.
“We begin from information labeling however then we type of develop and centralize a bunch of different information operations associated to coaching information,” says SuperAnnotate Co-founder and CEO Vahan Petrosyan. “The main focus continues to be the coaching information. However folks keep in our platform as a result of we handle that information effectively afterwards.”
For example, along with labeling and annotation, the SuperAnnotate product helps information engineers and information scientists discover information utilizing visualization instruments, construct CI/CD information orchestration pipelines for coaching information, generate artificial information, and consider how AI fashions carry out with sure information units. It helps to automate machine studying operations, or MLOps.
“The large worth that we now have is that we provide you with a bunch of various instruments to create a small subset of extremely curated, extremely correct information set to enhance massively your mannequin efficiency,” Petrosyan says.
Curating High quality Information
Vahan Petrosyan co-founded SuperAnnotate in 2018 along with his brother, Tigran Petrosyan. The Armenian brothers had been each PhD candidates at European universities, with Vahan finding out machine studying on the KTH Royal Institute of Know-how in Sweden and Tigran finding out physics on the College of Bern in Switzerland.
Vahan was creating a machine studying method at college that leveraged “tremendous pixels” for pc imaginative and prescient. As a substitute of constant along with his diploma, he determined to make use of the tremendous pixel discovery as the premise for an organization, dubbed SuperAnnotate, which they co-founded with two different engineers, Jason Liang and Davit Badalyan.
In January 2019, SuperAnnotate joined UC Berkeley’s SkyDeck accelerator program, and strikes its headquarters to Silicon Valley. After launching its first information annotation product in 2020, it raised greater than $17 million over the following 12 months and a half.
It concentrated its efforts on integration its information annotation platform with main information platforms, equivalent to Databricks, Snowflake, AWS, GCP, and Microsft Azure, to permit direct integration with the information.
When the generative AI revolution hit in late 2022, SuperAnnotate adopted its software program to help with fine-tuning of enormous language fashions (LLMs). Its been broadly adopted by some pretty giant firms, together with Nvidia, which was impressed sufficient with the product that it determined to turn out to be an investor with the November 20204 Collection B spherical that raised $36 million.
‘Evals Are All You Want’
One of many secrets and techniques to creating higher information for AI fashions–or what Petrosyan calls “tremendous information”–is having a well-defined and managed analysis course of. The eval course of, in flip, is crucial to enhancing AI efficiency over time utilizing reinforcement studying by way of human suggestions (RLHF).
One of the efficient eval methods entails creating extremely detailed question-answer pairs, Petrosyan says. These question-answer pairs instruct how the human information labelers and annotators ought to label and annotate the information to create the kind of AI that’s desired.
“People ought to collaborate with AI, no less than to judge the artificial information that’s being generated, to judge the question-answer pairs which are being written,” Petrosyan tells BigDATAwire. “And that information is turning into roughly the tremendous information that we’re discussing.”
By guiding how the information labeling and annotation is finished, the question-answer pairs permit organizations to fine-tune the conduct of black field AI fashions, with out altering any weights or parameters within the AI mannequin itself. These question-answer pairs can vary in size from a few pages to as much as 60 pages, and are crucial for addressing edge instances.
“In the event you’re Ford and also you’re deploying your chatbot, it shouldn’t actually say that Tesla is a greater automobile than Ford,” Petrosyan says. “And a few chatbots will say that. However you need to management all of that by simply offering examples, or labeling two completely different solutions, that that is the best way that I desire it to be answered in comparison with this different method, which says Tesla is a greater automobile than Ford.”
The eval step is a crucial however undervalued perform in AI, Petrosyan says. The OpenAI’s of the world perceive how worthwhile it may be to maintain feeding your AI with good, clear examples of the way you need the AI to behave, however many different gamers are lacking out on this necessary step.
“In the event you’re not very clear, there are tons of edge instances which are showing and so they’re producing a worse high quality information in consequence,” he says. “One of many co-founders of OpenAI [President Greg Brockman] stated evals are all you want to enhance the LLM mannequin.”
SuperAnnotate’s objectives is to assist prospects create higher information for AI, no more information. Information quantity isn’t a superb alternative for information high quality.
“Each small, tiny gadget is gathering a lot information that it’s virtually not helpful information,” Petrosyan says. “However how do you create clever information? That tremendous information goes to be your subsequent oil.”
Associated Objects:
Information At Extra Than Half Of Firms Will Not Be AI-Prepared By The Finish of 2024
To Forestall Generative AI Hallucinations and Bias, Combine Checks and Balances
The Prime 5 Information Labeling Companies In response to Everest Group