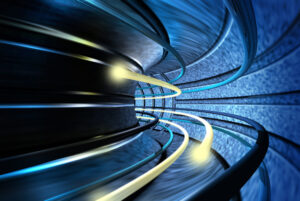
(anterovium/Shutterstock)
The power to harness, course of, and leverage huge quantities of information units main organizations aside in at present’s data-driven panorama. To remain forward, enterprises should grasp the complexities of synthetic intelligence (AI) information pipelines.
Using information analytics, BI functions, and information warehouses for structured information is a mature trade, and the methods to extract worth from structured information are well-known. Nonetheless, the rising explosion of generative AI now holds the promise of extracting hidden worth from unstructured information as properly. Enterprise information usually resides in disparate silos, every with its personal construction, format, and entry protocols. Integrating these various information sources is a major problem however an important first step in constructing an efficient AI information pipeline.
Within the quickly evolving panorama of AI, enterprises are continually striving to harness the complete potential of AI-driven insights. The spine of any profitable AI initiative is a sturdy information pipeline, which ensures that information flows seamlessly from supply to perception.
Overcoming Information Silo Obstacles to Speed up AI Pipeline Implementation
The limitations separating unstructured information silos have now turn out to be a extreme limitation to how rapidly IT organizations can implement AI pipelines with out prices, governance controls, and complexity spiraling uncontrolled.
Organizations want to have the ability to leverage their present information and might’t afford to overtake the prevailing infrastructure emigrate all their unstructured information to new platforms to implement AI methods. AI use circumstances and applied sciences are altering so quickly that information homeowners want the liberty to pivot at any time to scale up or down or to bridge a number of websites with their present infrastructure, all with out disrupting information entry for present customers or functions. As various because the AI use circumstances are, the widespread denominator amongst them is the necessity to gather information from many various sources and infrequently totally different areas.
The elemental problem is that entry to information by each people and AI fashions is at all times funneled by way of a file system in some unspecified time in the future – and file techniques have historically been embedded throughout the storage infrastructure. The results of this infrastructure-centric strategy is that when information outgrows the storage platform on which it resides, or if totally different efficiency necessities or price profiles dictate the usage of different storage varieties, customers and functions should navigate throughout a number of entry paths to incompatible techniques to get to their information.
This downside is especially acute for AI workloads, the place a crucial first step is consolidating information from a number of sources to allow a worldwide view throughout all of them. AI workloads should have entry to the entire dataset to categorise and/or label the recordsdata to find out which must be refined all the way down to the subsequent step within the course of.
With every section within the AI journey, the information can be refined additional. This refinement may embody cleaning and enormous language mannequin (LLM) coaching or, in some circumstances, tuning present LLMs for iterative inferencing runs to get nearer to the specified output. Every step additionally requires totally different compute and storage efficiency necessities, starting from slower, inexpensive mass storage techniques and archives, to high-performance and extra expensive NVMe storage.
The fragmentation brought on by the storage-centric lock-in of file techniques on the infrastructure layer just isn’t a brand new downside distinctive to AI use circumstances. For many years, IT professionals have been confronted with the selection of overprovisioning their storage infrastructure to resolve for the subset of information that wanted excessive efficiency or paying the “information copy tax” and added complexity to shuffle file copies between totally different techniques. This long-standing downside is now additionally evident within the coaching of AI fashions in addition to by way of the ETL course of.
Separating the File System from the Infrastructure Layer
Standard storage platforms embed the file system throughout the infrastructure layer. Nonetheless a software-defined resolution that’s appropriate with any on-premises or cloud-based storage platform from any vendor creates a high-performance, cross-platform Parallel International File System that spans incompatible storage silos throughout a number of areas.
With the file system decoupled from the underlying infrastructure, automated information orchestration supplies excessive efficiency to GPU clusters, AI fashions, and information engineers. All customers and functions in all areas have learn/write entry to all information in all places. To not file copies however to the identical recordsdata through this unified, international metadata management airplane.
Empowering IT Organizations with Self-Service Workflow Automation
Since many industries reminiscent of pharma, monetary providers, or biotechnology require each the archiving of coaching information in addition to the ensuing fashions, the power to automate the location of those information into low-cost sources is crucial. With customized metadata tags monitoring information provenance, iteration particulars, and different steps within the workflow, recalling outdated mannequin information for reuse or making use of a brand new algorithm is a straightforward operation that may be automated within the background.
The speedy shift to accommodate AI workloads has created a problem that exacerbates the silo issues that IT organizations have confronted for years. And the issues have been additive:
To be aggressive in addition to handle by way of the brand new AI workloads, information entry must be seamless throughout native silos, areas, and clouds, plus assist very high-performance workloads.
There’s a should be agile in a dynamic setting the place mounted infrastructure could also be troublesome to broaden because of price or logistics. In consequence, the power for firms to automate information orchestration throughout totally different siloed sources or quickly burst to cloud compute and storage sources has turn out to be important.
On the identical time, enterprises must bridge their present infrastructure with these new distributed sources cost-effectively and be certain that the price of implementing AI workloads doesn’t crush the anticipated return.
To maintain up with the numerous efficiency necessities for AI pipelines, a brand new paradigm is important that might successfully bridge the gaps between on-premises silos and the cloud. Such an answer requires new expertise and a revolutionary strategy to elevate the file system out of the infrastructure layer to allow AI pipelines to make the most of present infrastructure from any vendor with out compromising outcomes.
Concerning the creator: Molly Presley brings over 15 years of product and progress advertising and marketing management expertise to the Hammerspace workforce. Molly has led the advertising and marketing group and technique at fast-growth innovators reminiscent of Pantheon Platform, Qumulo, Quantum Company, DataDirect Networks (DDN), and Spectra Logic. She was liable for the go-to-market technique for SaaS, hybrid cloud, and information middle options throughout numerous data-intensive verticals and use circumstances in these firms. At Hammerspace, Molly leads the advertising and marketing group and evokes information creators and customers to take full benefit of a really international information setting.
Associated Gadgets:
Three Methods to Join the Dots in a Decentralized Massive Information World
Object Storage a ‘Whole Cop Out,’ Hammerspace CEO Says. ‘You All Obtained Duped’
Hammerspace Hits the Market with International Parallel File System