What an unbelievable week we’ve already had at re:Invent 2023! Should you haven’t checked them out already, I encourage you to learn our staff’s weblog posts masking Monday Night time Dwell with Peter DeSantis and Tuesday’s keynote from Adam Selipsky.
Immediately we heard Dr. Swami Sivasubramanian’s keynote deal with at re:Invent 2023. Dr. Sivasubramanian is the Vice President of Information and AI at AWS. Now greater than ever, with the current proliferation of generative AI providers and choices, this house is ripe for innovation and new service releases. Let’s see what this yr has in retailer!
Swami started his keynote by outlining how over 200 years of technological innovation and progress within the fields of mathematical computation, new architectures and algorithms, and new programming languages has led us to this present inflection level with generative AI. He challenged everybody to have a look at the alternatives that generative AI presents when it comes to intelligence augmentation. By combining information with generative AI, collectively in a symbiotic relationship with human beings, we will speed up new improvements and unleash our creativity.
Every of at this time’s bulletins could be considered by way of the lens of a number of of the core components of this symbiotic relationship between information, generative AI, and people. To that finish, Swami supplied a listing of the next necessities for constructing a generative AI software:
- Entry to a wide range of basis fashions
- Non-public atmosphere to leverage your information
- Straightforward-to-use instruments to construct and deploy purposes
- Function-built ML infrastructure
On this put up, I can be highlighting the principle bulletins from Swami’s keynote, together with:
- Help for Anthropic’s Claude 2.1 basis mannequin in Amazon Bedrock
- Amazon Titan Multimodal Embeddings, Textual content fashions, and Picture Generator now obtainable in Amazon Bedrock
- Amazon SageMaker HyperPod
- Vector engine for Amazon OpenSearch Serverless
- Vector seek for Amazon DocumentDB (with MongoDB compatibility) and Amazon MemoryDB for Redis
- Amazon Neptune Analytics
- Amazon OpenSearch Service zero-ETL integration with Amazon S3
- AWS Clear Rooms ML
- New AI capabilities in Amazon Redshift
- Amazon Q generative SQL in Amazon Redshift
- Amazon Q information integration in AWS Glue
- Mannequin Analysis on Amazon Bedrock
Let’s start by discussing a few of the new basis fashions now obtainable in Amazon Bedrock!
Anthropic Claude 2.1
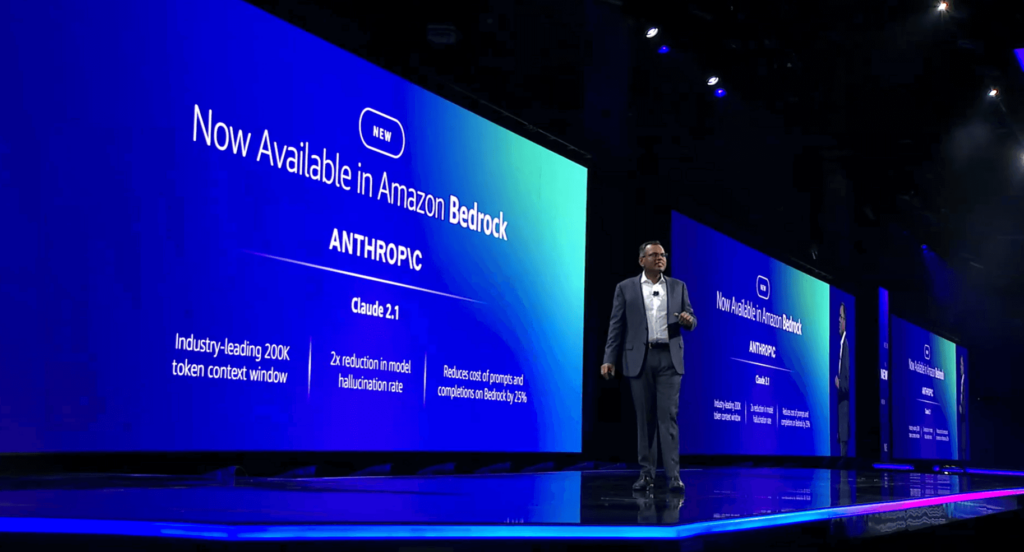
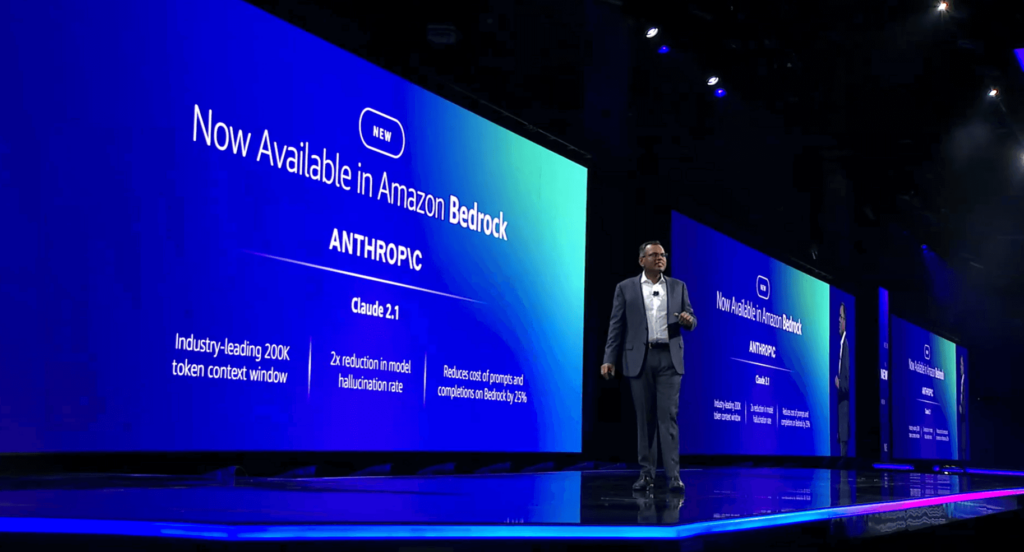
Simply final week, Anthropic introduced the discharge of its newest mannequin, Claude 2.1. Immediately, this mannequin is now obtainable inside Amazon Bedrock. It presents vital advantages over prior variations of Claude, together with:
- A 200,000 token context window
- A 2x discount within the mannequin hallucination charge
- A 25% discount in the price of prompts and completions on Bedrock
These enhancements assist to reinforce the reliability and trustworthiness of generative AI purposes constructed on Bedrock. Swami additionally famous how accessing a wide range of basis fashions (FMs) is important and that “nobody mannequin will rule all of them.” To that finish, Bedrock presents assist for a broad vary of FMs, together with Meta’s Llama 2 70B, which was additionally introduced at this time.
Amazon Titan Multimodal Embeddings, Textual content fashions, and Picture Generator now obtainable in Amazon Bedrock


Swami launched the idea of vector embeddings, that are numerical representations of textual content. These embeddings are vital when customizing and enhancing generative AI purposes with issues like multimodal search, which may contain a text-based question together with uploaded photographs, video, or audio. To that finish, he launched Amazon Titan Multimodal Embeddings, which may settle for textual content, photographs, or a mix of each to supply search, advice, and personalization capabilities inside generative AI purposes. He then demonstrated an instance software that leverages multimodal search to help prospects find the required instruments and sources to finish a family reworking undertaking primarily based on a consumer’s textual content enter and image-based design selections.
He additionally introduced the final availability of Amazon Titan Textual content Lite and Amazon Titan Textual content Categorical. Titan Textual content Lite is helpful for performing duties like summarizing textual content and copywriting, whereas Titan Textual content Categorical can be utilized for open-ended textual content era and conversational chat. Titan Textual content Categorical additionally helps retrieval-augmented era, or RAG, which is helpful when coaching your personal FMs primarily based in your group’s information.
He then launched Titan Picture Generator and confirmed how it may be used to each generate new photographs from scratch and edit current photographs primarily based on pure language prompts. Titan Picture Generator additionally helps the accountable use of AI by embedding an invisible watermark inside each picture it generates indicating that the picture was generated by AI.
Amazon SageMaker HyperPod
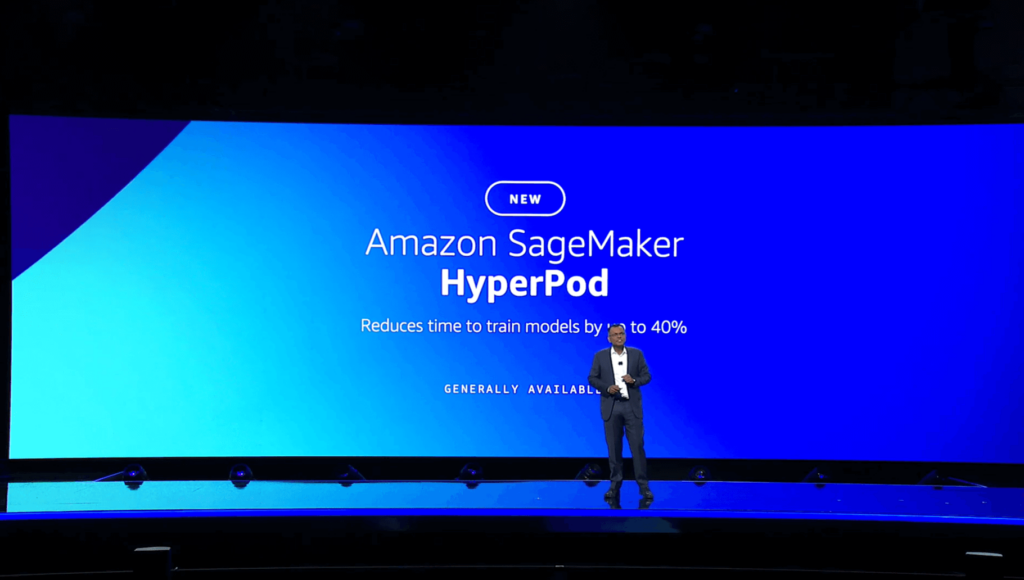
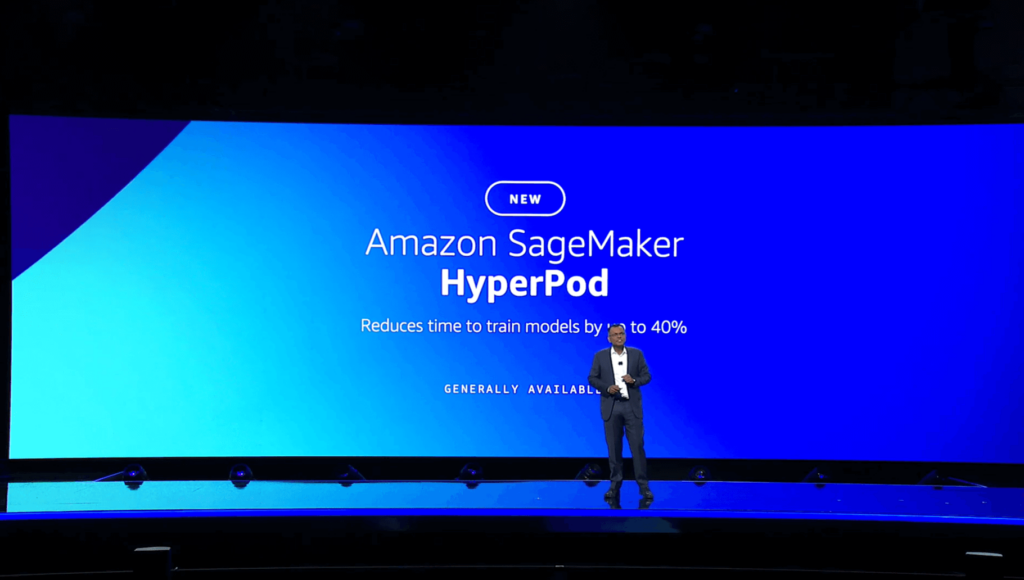
Swami then moved on to a dialogue concerning the complexities and challenges confronted by organizations when coaching their very own FMs. These embody needing to interrupt up giant datasets into chunks which can be then unfold throughout nodes inside a coaching cluster. It’s additionally essential to implement checkpoints alongside the best way to protect towards information loss from a node failure, including additional delays to an already time and resource-intensive course of. SageMaker HyperPod reduces the time required to coach FMs by permitting you to separate your coaching information and mannequin throughout resilient nodes, permitting you to coach FMs for months at a time whereas taking full benefit of your cluster’s compute and community infrastructure, lowering the time required to coach fashions by as much as 40%.
Vector engine for Amazon OpenSearch Serverless


Returning to the topic of vectors, Swami defined the necessity for a robust information basis that’s complete, built-in, and ruled when constructing generative AI purposes. In assist of this effort, AWS has developed a set of providers on your group’s information basis that features investments in storing vectors and information collectively in an built-in vogue. This lets you use acquainted instruments, keep away from further licensing and administration necessities, present a quicker expertise to finish customers, and scale back the necessity for information motion and synchronization. AWS is investing closely in enabling vector search throughout all of its providers. The primary announcement associated to this funding is the final availability of the vector engine for Amazon OpenSearch Serverless, which lets you retailer and question embeddings immediately alongside your corporation information, enabling extra related similarity searches whereas additionally offering a 20x enchancment in queries per second, all with no need to fret about sustaining a separate underlying vector database.
Vector seek for Amazon DocumentDB (with MongoDB compatibility) and Amazon MemoryDB for Redis
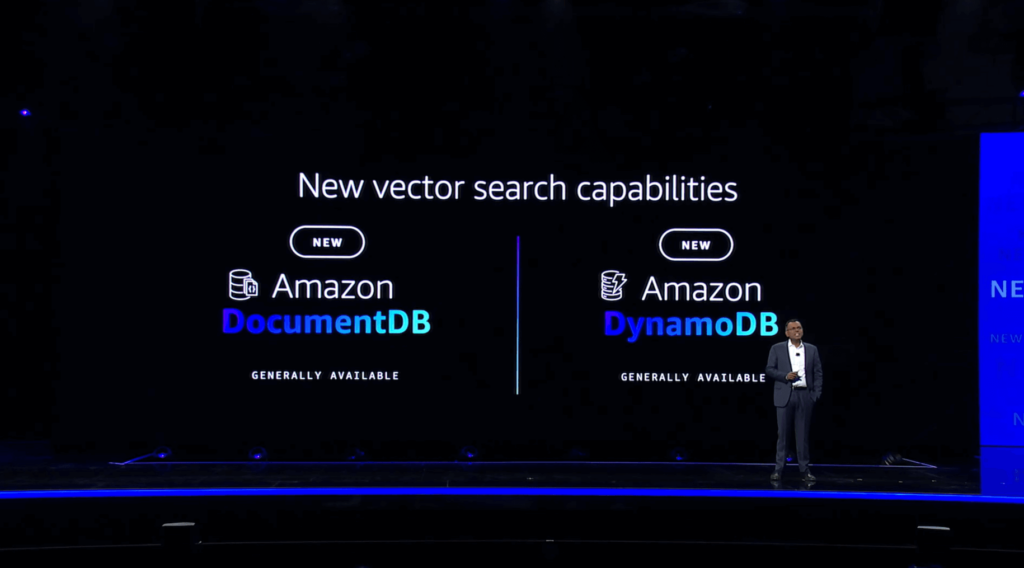
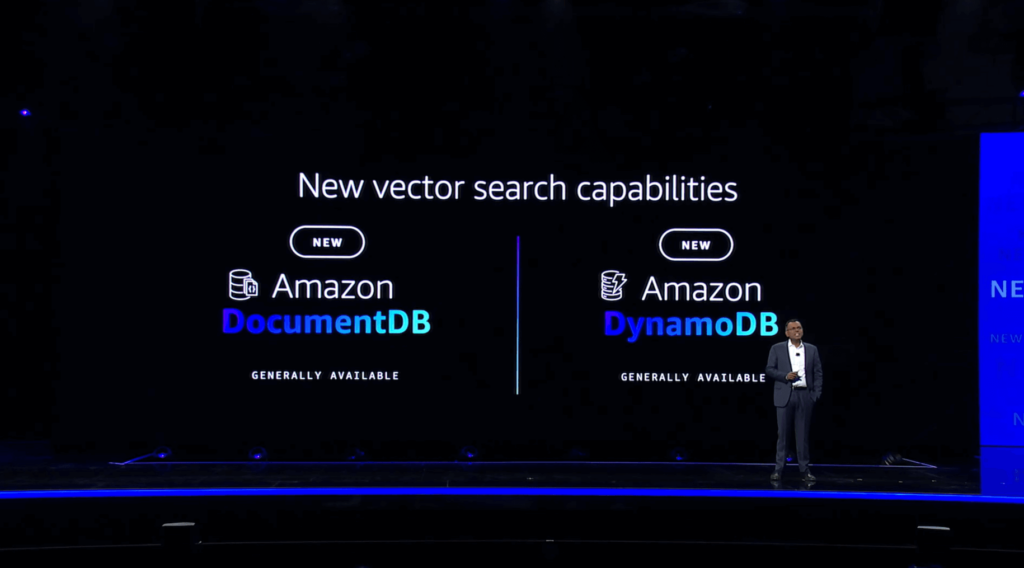
Vector search capabilities had been additionally introduced for Amazon DocumentDB (with MongoDB compatibility) and Amazon MemoryDB for Redis, becoming a member of their current providing of vector search inside DynamoDB. These vector search choices all present assist for each excessive throughput and excessive recall, with millisecond response instances even at concurrency charges of tens of 1000’s of queries per second. This degree of efficiency is particularly essential inside purposes involving fraud detection or interactive chatbots, the place any diploma of delay could also be pricey.
Amazon Neptune Analytics
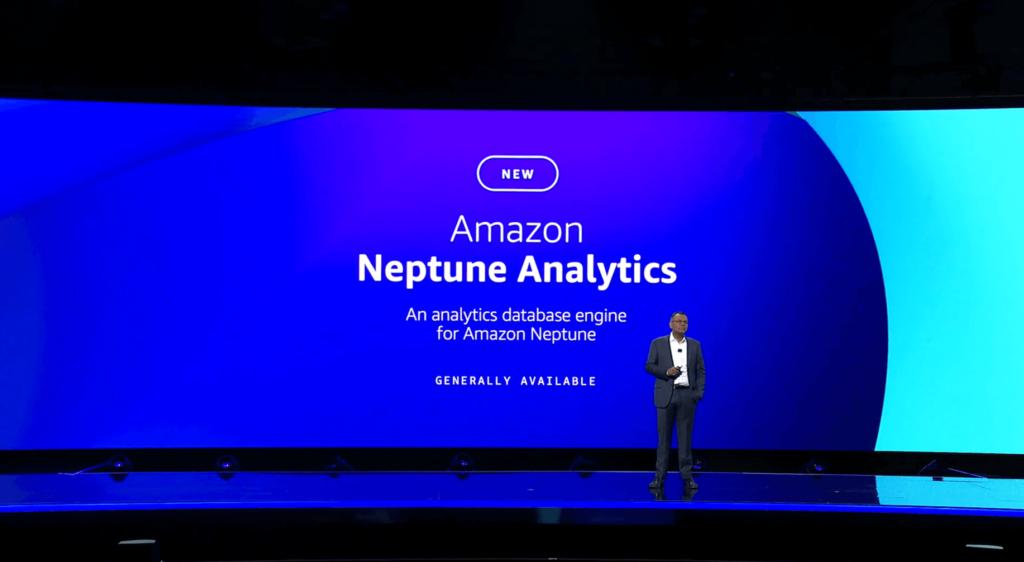
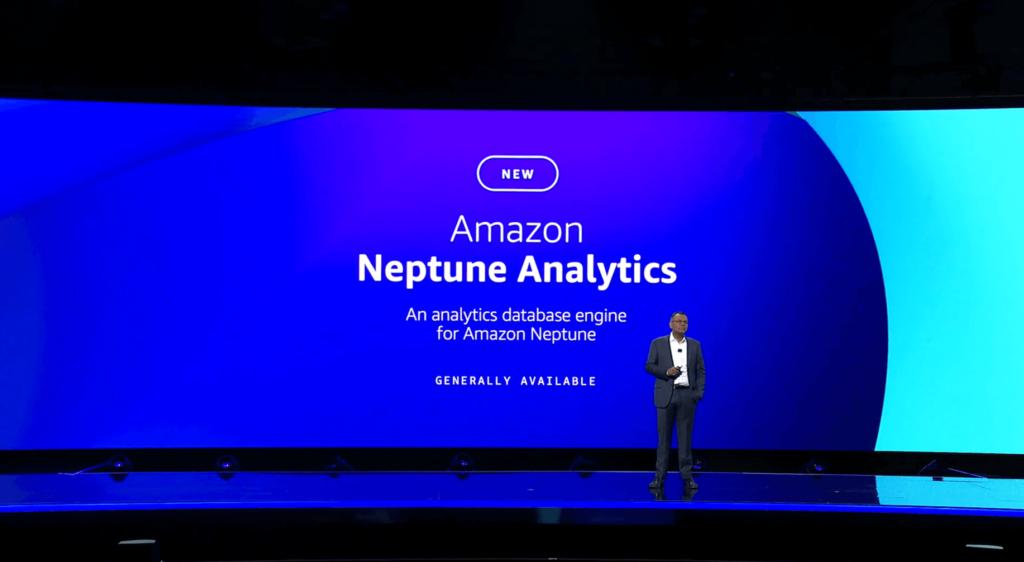
Staying inside the realm of AWS database providers, the subsequent announcement centered round Amazon Neptune, a graph database that permits you to signify relationships and connections between information entities. Immediately’s announcement of the final availability of Amazon Neptune Analytics makes it quicker and simpler for information scientists to rapidly analyze giant volumes of information saved inside Neptune. Very like the opposite vector search capabilities talked about above, Neptune Analytics allows quicker vector looking by storing your graph and vector information collectively. This lets you discover and unlock insights inside your graph information as much as 80x quicker than with current AWS options by analyzing tens of billions of connections inside seconds utilizing built-in graph algorithms.
Amazon OpenSearch Service zero-ETL integration with Amazon S3
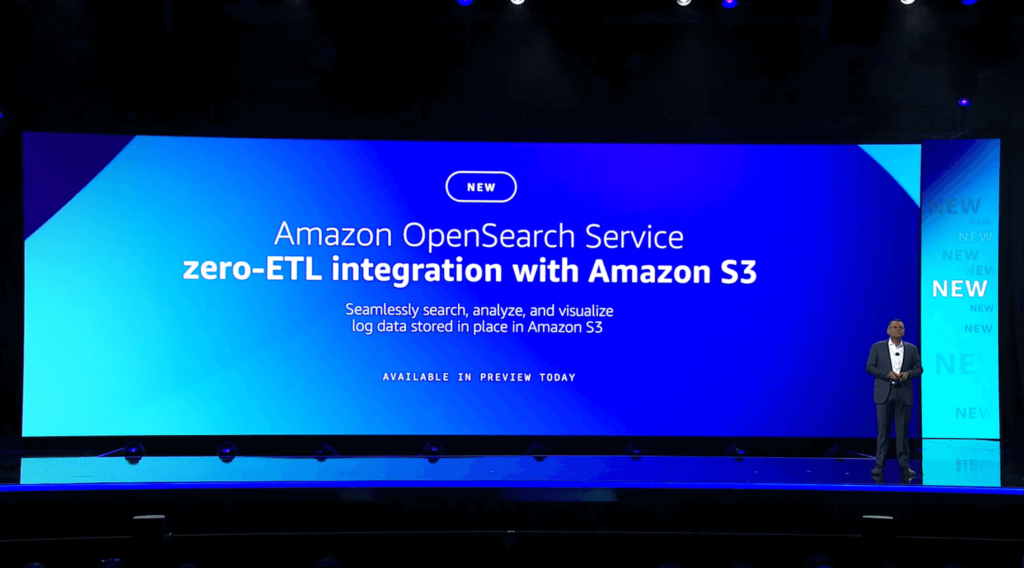
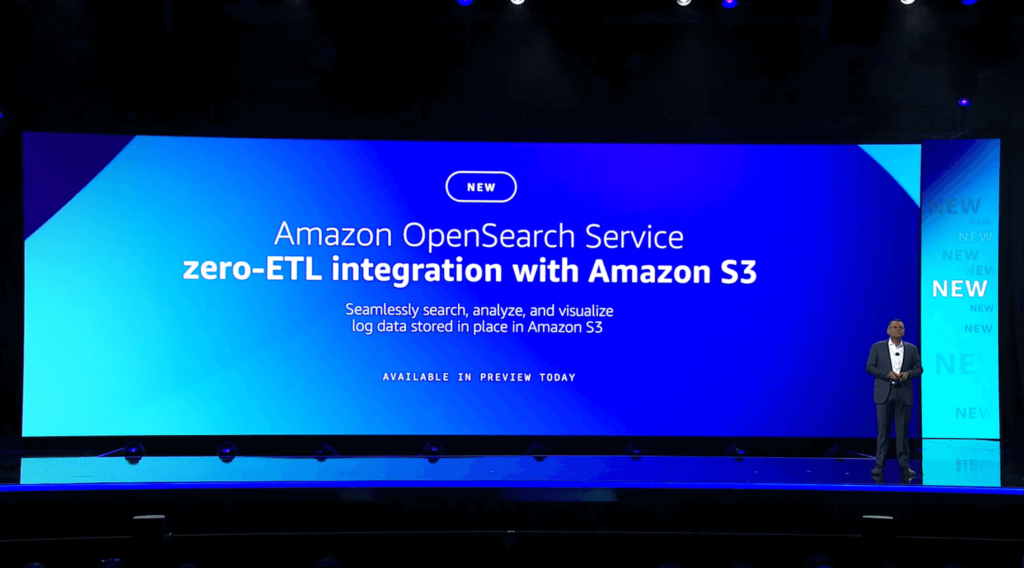
Along with enabling vector search throughout AWS database providers, Swami additionally outlined AWS’ dedication to a “zero-ETL” future, with out the necessity for sophisticated and costly extract, rework, and cargo, or ETL pipeline growth. AWS has already introduced quite a few new zero-ETL integrations this week, together with Amazon DynamoDB zero-ETL integration with Amazon OpenSearch Service and numerous zero-ETL integrations with Amazon Redshift. Immediately, Swami introduced one other new zero-ETL integration, this time between Amazon OpenSearch Service and Amazon S3. Now obtainable in preview, this integration permits you to seamlessly search, analyze, and visualize your operational information saved in S3, akin to VPC Movement Logs and Elastic Load Balancing logs, in addition to S3-based information lakes. You’ll additionally be capable of leverage OpenSearch’s out of the field dashboards and visualizations.
AWS Clear Rooms ML


Swami went on to debate AWS Clear Rooms, which had been launched earlier this yr and permit AWS prospects to securely collaborate with companions in “clear rooms” that don’t require you to repeat or share any of your underlying uncooked information. Immediately, AWS introduced a preview launch of AWS Clear Rooms ML, extending the clear rooms paradigm to incorporate collaboration on machine studying fashions by way of using AWS-managed lookalike fashions. This lets you practice your personal customized fashions and work with companions with no need to share any of your personal uncooked information. AWS additionally plans to launch a healthcare mannequin to be used inside Clear Rooms ML inside the subsequent few months.
New AI capabilities in Amazon Redshift
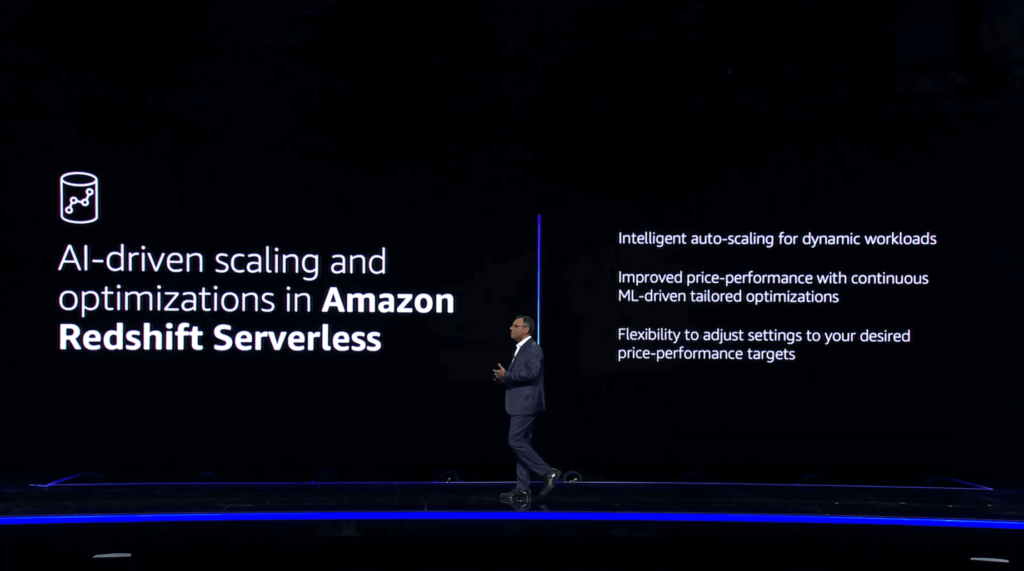
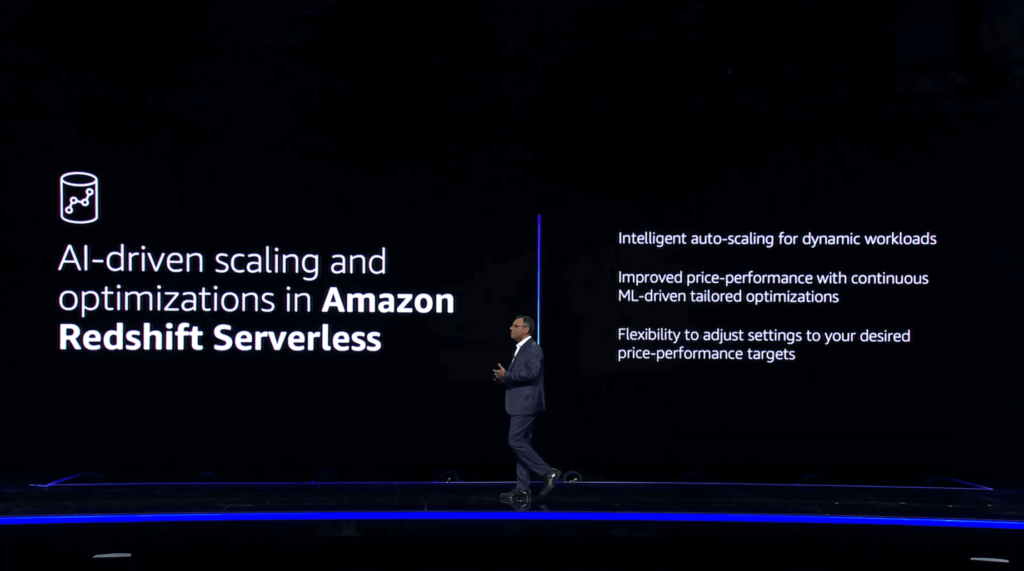
The subsequent two bulletins each contain Amazon Redshift, starting with some AI-driven scaling and optimizations in Amazon Redshift Serverless. These enhancements embody clever auto-scaling for dynamic workloads, which presents proactive scaling primarily based on utilization patterns that embody the complexity and frequency of your queries together with the dimensions of your information units. This lets you give attention to deriving essential insights out of your information moderately than worrying about efficiency tuning your information warehouse. You may set price-performance targets and reap the benefits of ML-driven tailor-made optimizations that may do the whole lot from adjusting your compute to modifying the underlying schema of your database, permitting you to optimize for value, efficiency, or a stability between the 2 primarily based in your necessities.
Amazon Q generative SQL in Amazon Redshift


The subsequent Redshift announcement is unquestionably certainly one of my favorites. Following yesterday’s bulletins about Amazon Q, Amazon’s new generative AI-powered assistant that may be tailor-made to your particular enterprise wants and information, at this time we realized about Amazon Q generative SQL in Amazon Redshift. Very like the “pure language to code” capabilities of Amazon Q that had been unveiled yesterday with Amazon Q Code Transformation, Amazon Q generative SQL in Amazon Redshift permits you to write pure language queries towards information that’s saved in Redshift. Amazon Q makes use of contextual details about your database, its schema, and any question historical past towards your database to generate the required SQL queries primarily based in your request. You may even configure Amazon Q to leverage the question historical past of different customers inside your AWS account when producing SQL. It’s also possible to ask questions of your information, akin to “what was the highest promoting merchandise in October” or “present me the 5 highest rated merchandise in our catalog,” with no need to know your underlying desk construction, schema, or any sophisticated SQL syntax.
Amazon Q information integration in AWS Glue
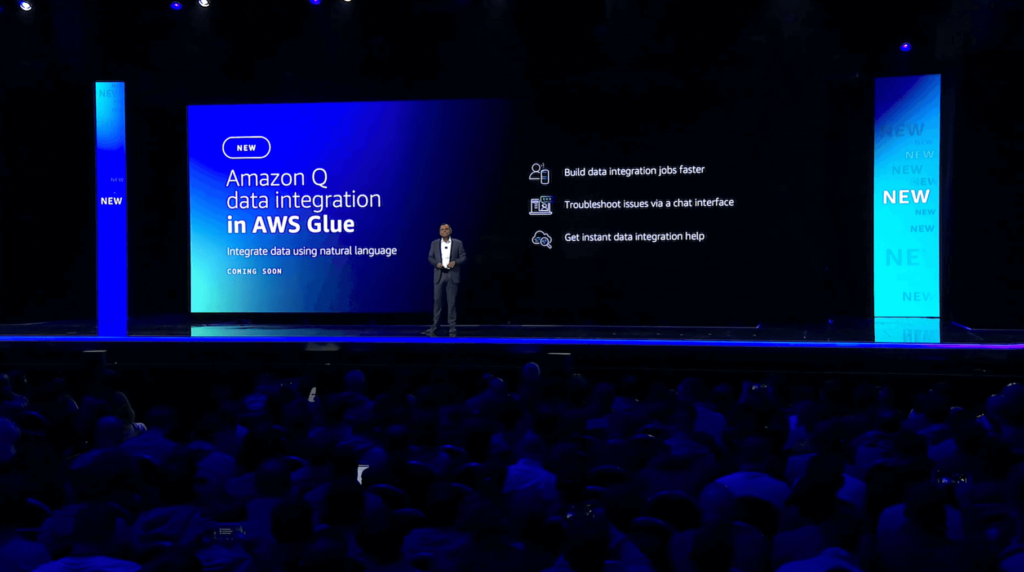
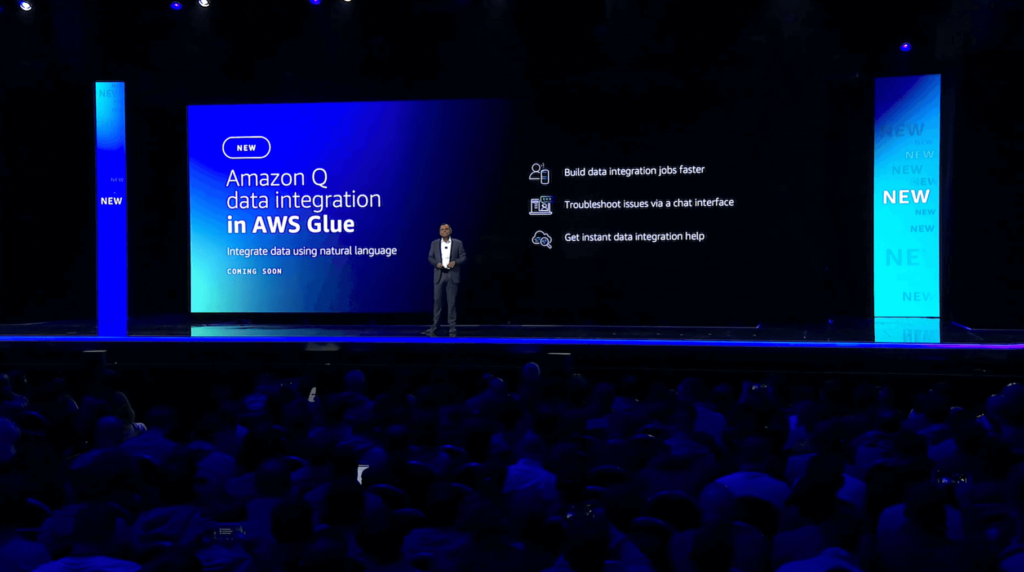
One further Amazon Q-related announcement concerned an upcoming information integration in AWS Glue. This promising characteristic will simplify the method of developing customized ETL pipelines in eventualities the place AWS doesn’t but supply a zero-ETL integration, leveraging brokers for Amazon Bedrock to interrupt down a pure language immediate right into a collection of duties. As an example, you might ask Amazon Q to “write a Glue ETL job that reads information from S3, removes all null information, and masses the information into Redshift” and it’ll deal with the remainder for you robotically.
Mannequin Analysis on Amazon Bedrock
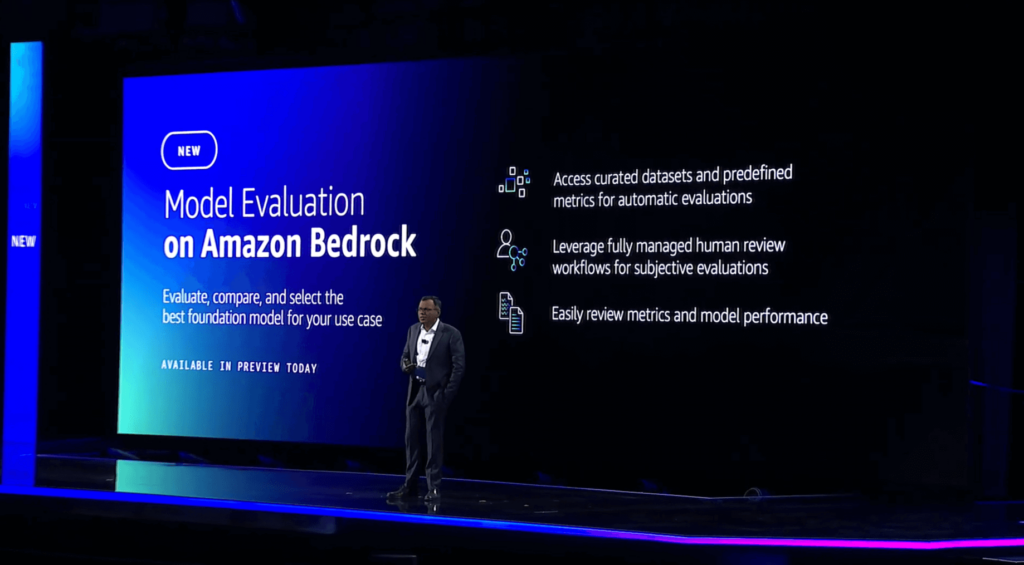
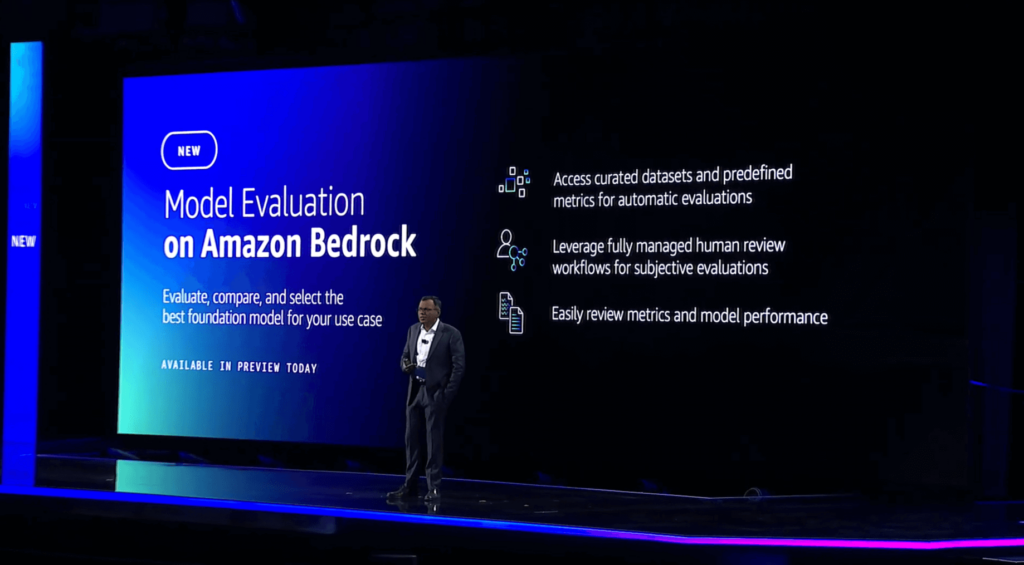
Swami’s closing announcement circled again to the number of basis fashions which can be obtainable inside Amazon Bedrock and his earlier assertion that “nobody mannequin will rule all of them.” Due to this, mannequin evaluations are an essential device that must be carried out steadily by generative AI software builders. Immediately’s preview launch of Mannequin Analysis on Amazon Bedrock permits you to consider, examine, and choose the most effective FM on your use case. You may select to make use of automated analysis primarily based on metrics akin to accuracy and toxicity, or human analysis for issues like fashion and applicable “model voice.” As soon as an analysis job is full, Mannequin Analysis will produce a mannequin analysis report that comprises a abstract of metrics detailing the mannequin’s efficiency.
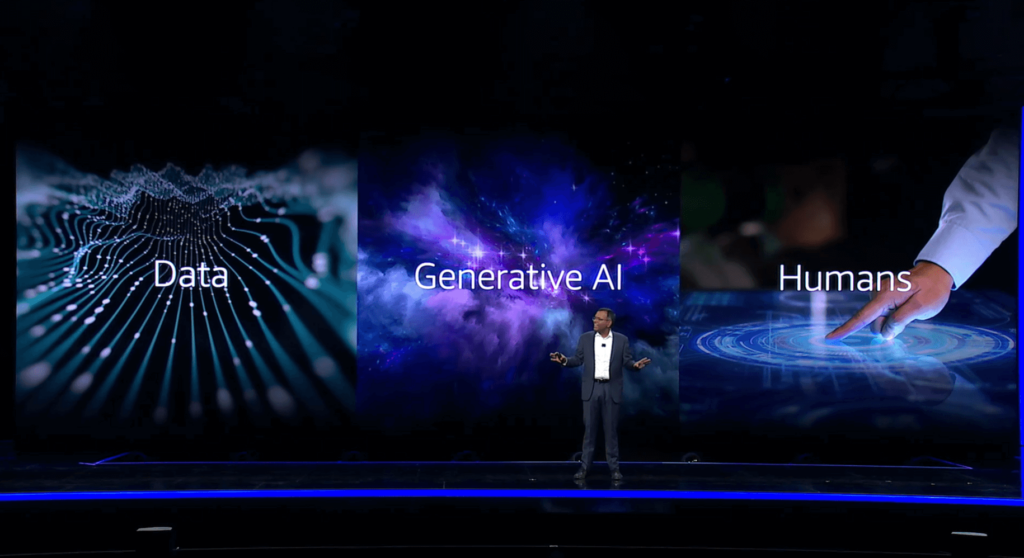
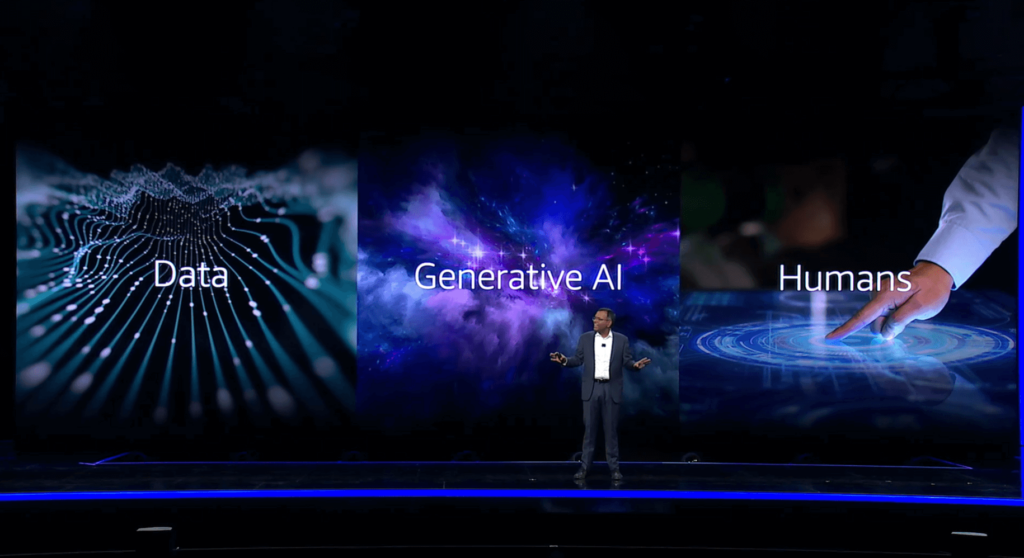
Swami concluded his keynote by addressing the human factor of generative AI and reaffirming his perception that generative AI purposes will speed up human productiveness. In any case, it’s people who should present the important inputs essential for generative AI purposes to be helpful and related. The symbiotic relationship between information, generative AI, and people creates longevity, with collaboration strengthening every factor over time. He concluded by asserting that people can leverage information and generative AI to “create a flywheel of success.” With the upcoming generative AI revolution, human smooth expertise akin to creativity, ethics, and flexibility can be extra essential than ever. In response to a World Financial Discussion board survey, practically 75% of corporations will undertake generative AI by the yr 2027. Whereas generative AI might get rid of the necessity for some roles, numerous new roles and alternatives will little doubt emerge within the years to return.
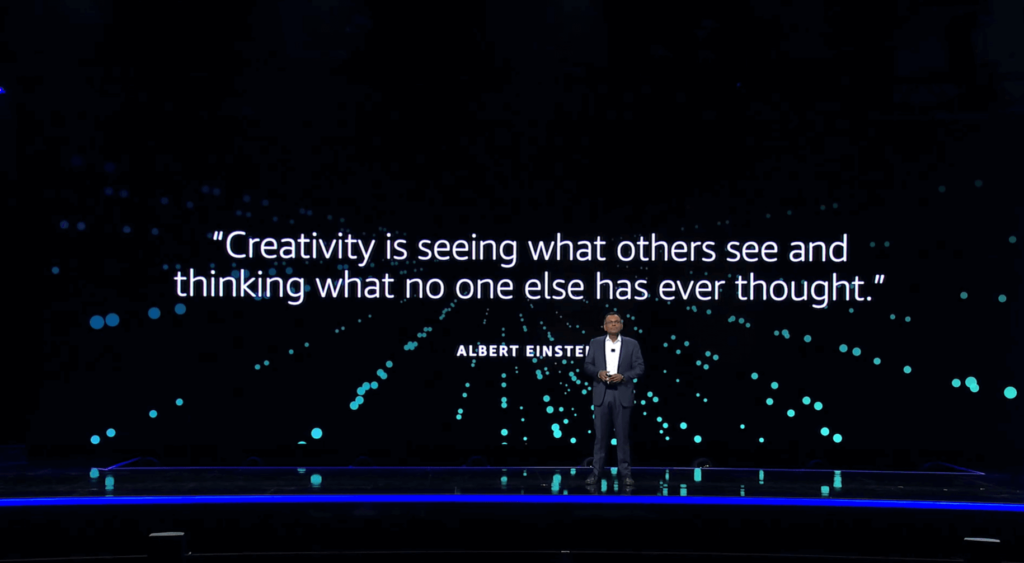
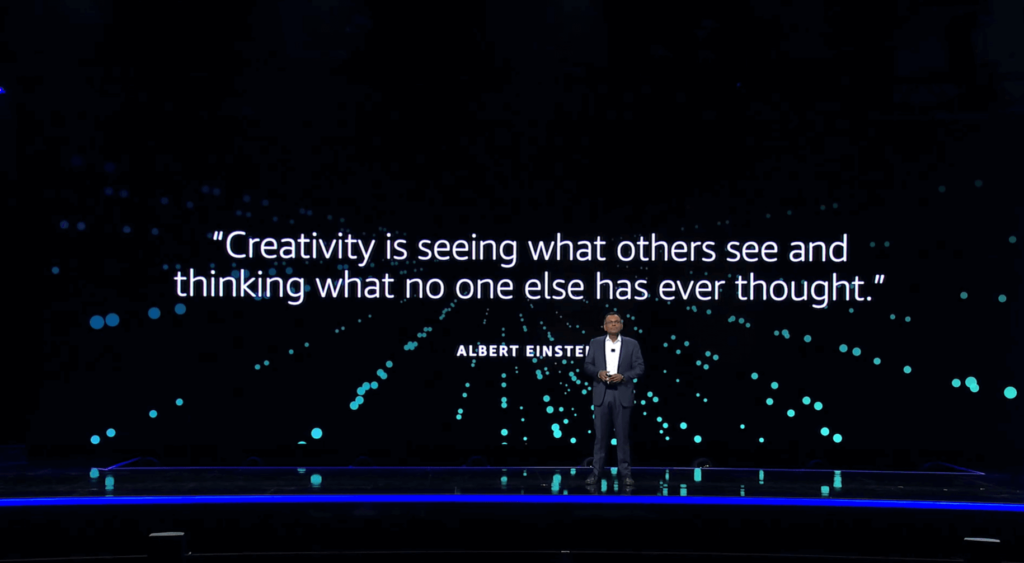
I entered at this time’s keynote full of pleasure and anticipation, and as standard, Swami didn’t disappoint. I’ve been totally impressed by the breadth and depth of bulletins and new characteristic releases already this week, and it’s solely Wednesday! Keep watch over our weblog for extra thrilling keynote bulletins from re:Invent 2023!