Introduction
Equiniti (EQ), a world chief in shareholder, pension, and remediation providers, leveraged Databricks to revolutionize its data-driven method and improve buyer expertise throughout 136 international locations. Serving over 6,000 corporations, EQ acknowledged the necessity to adapt to more and more complicated and controlled environments by harnessing the ability of superior analytics and generative AI.
Provided that well timed entry to data is vital to Equiniti’s clients’ success, they needed to make information (and data-driven insights) the inspiration of their operational and strategic method. Equiniti aimed to implement extra knowledgeable, environment friendly and efficient enterprise practices and make the most of new developments in superior analytics and GenAI that will improve buyer expertise and drive inside innovation.
To satisfy these targets, Equiniti wanted to construct a future-proof, safe and performant information platform that might assist any present or new information and AI functions. This weblog describes how and why they chose Databricks Knowledge Intelligence Platform to assist their infrastructure and elaborates on the superior use instances they’ve already explored by leveraging the Databricks Platform and Databricks Mosaic AI instruments, corresponding to the event of PensionGuru, their GenAI-powered chatbot.
Step 1: Establish worth and construct strong information foundations
Relatively than beginning with the query, ‘What can we use AI for?’, Equiniti requested, ‘How can we offer new worth to our shoppers, utilizing high-quality, trusted information and trendy instruments and strategies?’
Just a few frequent themes emerged: having access to trusted information at scale, having the agility to experiment and transfer shortly and cost-effectively, expediting the enablement of area subject material consultants (SMEs) and present sources, and having the ability to shortly modernize their choices to fulfill shopper wants.
From that preliminary work, Equiniti recognized key necessities for a future cloud information and AI platform that will allow them to greatest unlock the worth of their information:
● Built-in information and AI governance: With out governance and management, there might be no worth. Equiniti wanted sturdy safety features, entry controls, computerized lineage and auditing that will assist keep compliance with regulatory necessities by monitoring the move and transformation of information throughout the platform and construct belief with inside and exterior stakeholders and shoppers.
● A unified and open platform: One other requirement was a easy structure that might assist information engineering, information science, superior analytics, and GenAI. Equiniti needed to remove silos and pointless information duplications and keep away from being locked right into a proprietary resolution. They needed a platform that was constructed on open requirements and protocols. As well as, they wanted assist for each batch and streaming information sources in any format for GenAI workloads. With the distributed nature of their information and programs, a single platform that might turn into an analytical supply of reality could be an enormous step ahead.
● Value optimization: Lastly, Equinity wanted scalable and optimized compute that enhanced information processing and lowered TCO with a real consumption-based mannequin. The flexibility to start with a low preliminary funding after which scale as wanted was important.
With these necessities in thoughts, Equiniti selected the Databricks Knowledge Intelligence Platform because the spine of their trendy cloud information and AI platform.
Step 2: Transfer quick and leverage built-in toolsets
Historically, it takes enter from many alternative groups to guage separate elements and distinct providers that kind a knowledge platform, requiring the navigation of competing priorities and sources to implement it. Nevertheless, Equiniti was capable of shortly and simply deploy the Databricks Platform and discover all of its built-in capabilities. The choice to experiment and scale shortly however cost-effectively meant that Equiniti might confidently make selections in prototyping connectivity, information processing and analytical capabilities with out vital up-front funding in time or price. As soon as Equiniti established the first use instances for his or her preliminary AI implementation, they collaborated with the Databricks workforce to create an preliminary structure, as proven in Determine 1 under. By a set of workshops, Databricks resolution architects showcased learn how to greatest make the most of the built-in capabilities of the platform; Equiniti additionally used complete self-paced studying sources to upskill themselves.
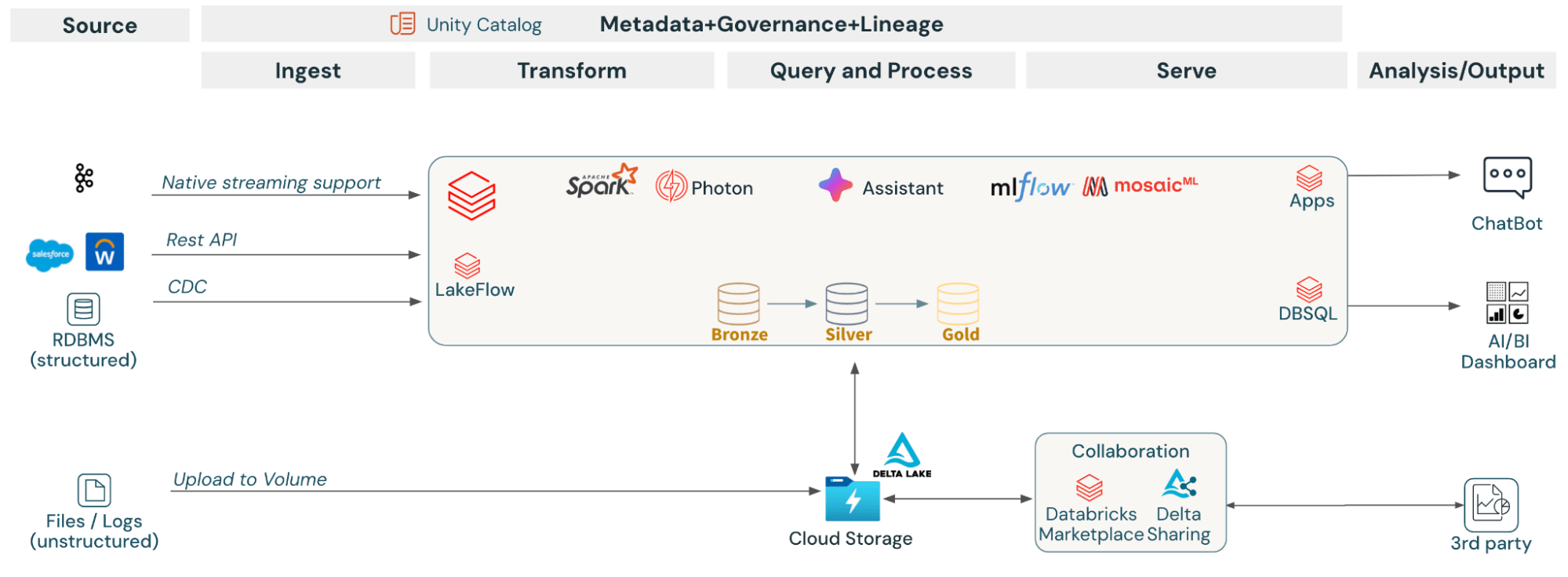
One of the crucial priceless options of the Databricks Platform is Unity Catalog, a unified and open governance resolution for information and AI. The flexibility to trace the robotically captured lineage of the ingested information and the way it was reworked and used within the mannequin was key to constructing belief, understanding, and approval from Equiniti’s InfoSec and Danger groups. Equiniti was capable of display what and the place information was getting used, with out further price, implementation overhead and time in managing a separate information catalog. As well as, Delta Sharing and Databricks Market have been transformational, as they allowed Equiniti to externally share information with companions for the very first time. Gaining the power to visualise information from a number of sources that have been beforehand inaccessible or siloed and using information from exterior suppliers with out having to retailer and keep petabyte-scale datasets has allowed Equiniti to shortly and simply develop insights that have been beforehand out of attain. The flexibility for enterprise groups to simply uncover and use the identical instruments and information property from a central, trusted supply will proceed to drive high quality and worth of their information platform.
For Equiniti’s small engineering workforce, one of many largest time-saving options of the Databricks Platform was LakeFlow Join. Databricks LakeFlow offers built-in connectors for ingesting information from enterprise functions and databases. The flexibility to seamlessly create no-code integrations to our core platforms corresponding to Workday, Salesforce and SQL Server massively lowered the time it took to make information out there in Databricks for fashions to eat. It considerably lowered storage and compute prices and saved Equiniti months of growth work in comparison with the normal technique of constructing API integrations and ETL processes to retailer and handle information. Equiniti’s workforce might then give attention to worth multiplier areas corresponding to creating Gen AI functions that will ship worth to the enterprise.
Lastly, growing these new GenAI functions requires a brand new kind of “information developer.” These are area SMEs who deeply perceive the enterprise (in Equiniti’s case, the pensions market). These consultants should have seamless entry to instruments and platforms to supply essential suggestions and ensure GenAI functions are delivering correct and high-quality outcomes. The convenience of use and accessibility of the Databricks Platform platform made it simple for SMEs to successfully collaborate with the event and engineering groups in constructing GenAI functions. By leveraging their experience and deep enterprise insights, Equiniti was capable of set up floor reality and obtain priceless suggestions, which helped fine-tune responses and generated content material to be used throughout the group.
Step 3: Present worth, ship outcomes, and maintain innovating
Considered one of Equiniti’s first GenAI use instances was the event of their GenAI chatbot, PensionGuru. Given Equiniti’s function in administering quite a few pension plans, its workers typically have to navigate and interpret an intensive quantity of paperwork, together with insurance policies, belief deeds, and tips. PensionGuru addresses this problem by providing prompt, correct responses, streamlining entry to complicated data and bettering productiveness.
The app considerably boosts enterprise effectivity by automating doc evaluation and minimizing the time required to extract vital particulars, thereby decreasing administrative overhead. Duties that have been taking many hours previously are actually accomplished in minutes. PensionGuru empowers workers to shortly and precisely retrieve data, bettering service supply and decision-making processes. By using superior pure language processing, the app understands and processes consumer queries intelligently, delivering contextually related data from huge datasets. This innovation not solely saves time but in addition enhances data-driven insights, permitting for a extra strategic method to pension scheme administration.
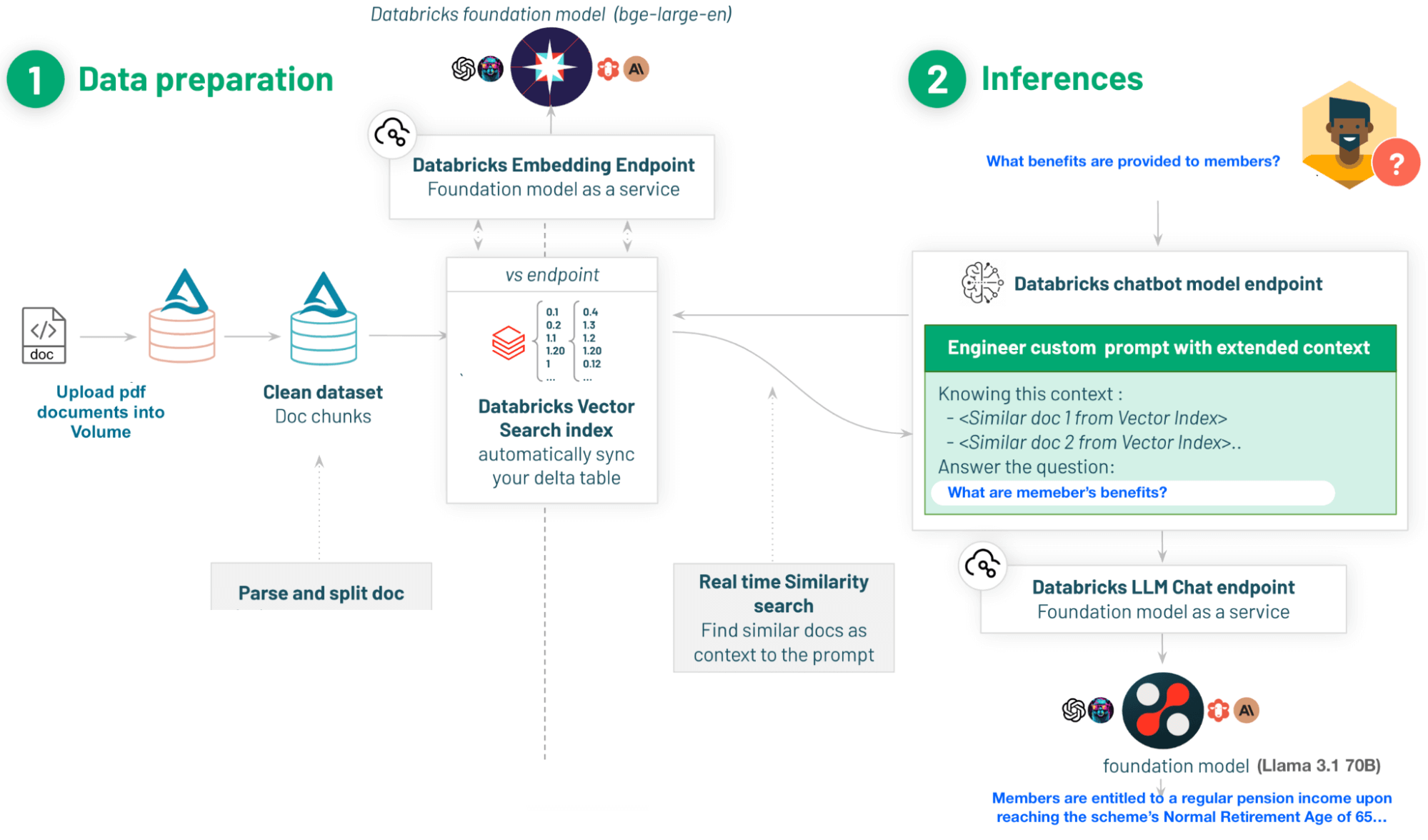
To create PensionGuru, Equiniti started by taking hundreds of pension paperwork, initially saved as PDF recordsdata, and loading them right into a Databricks Quantity, as proven in Determine 2 above. Then, Equiniti effectively managed these unstructured recordsdata by Unity Catalog, proper from the purpose of ingestion. The subsequent step was to extract textual content from the paperwork, divided it into manageable chunks, and retailer the information in a Delta Desk. Utilizing Mosaic AI Vector Search with a serverless setup, Equiniti simply constructed a vector database to assist search and retrieval capabilities.
To energy the appliance, Equiniti leveraged Mosaic AI Mannequin Serving to ascertain an LLM endpoint primarily based on the highly effective and cost-effective open supply Meta Llama 3.1 70B mannequin. Lastly, Equiniti was capable of seamlessly and securely deploy the chatbot to finish customers with Databricks Apps, a brand new easy and serverless resolution for creating production-ready apps with built-in governance on high of the Databricks Knowledge Intelligence Platform. The built-in Apps characteristic was an enormous time saver and a large sport changer, because it eliminated the necessity for Equiniti’s information workforce to deploy, handle and keep the underlying infrastructure to assist the appliance. The workforce might as an alternative give attention to delivering enterprise worth as an alternative of spending time on mundane duties like siloed providers integration and IT infrastructure administration.
The preliminary PensionGuru outcomes and suggestions have been extremely encouraging, and Equiniti continues to refine and improve the appliance’s efficiency by ongoing experimentation and mannequin coaching. They’re additionally exploring the incorporation of an AI agent framework that will permit them to additional customise and prolong the capabilities of PensionGuru, making it much more responsive and tailor-made to the precise wants of pension scheme administration. With this method, Equiniti goals to ship even larger accuracy and effectivity in processing and retrieving vital pension data.
Conclusion
By deciding on the Databricks Knowledge Intelligence Platform, Equiniti has delivered an answer that’s modular, extensible and able to assembly all present and future information and AI wants. Databricks’ means to unify information engineering, information science, machine studying, and GenAI right into a single resolution permits Equiniti to realize excessive ranges of effectivity and scalability. This complete method is anchored across the foundational information governance with Unity Catalog, which promotes information accessibility throughout the group.
Moreover, the Databricks Platform’s superior instruments and environments for AI mannequin growth and deployment have unlocked new alternatives, fueling each innovation and operational effectivity with out sacrificing information integration, safety and governance.
“Though we’re early on our Generative AI journey, we’re assured in our means to ship significant enterprise worth with the Databricks Knowledge Intelligence Platform.”
— James West, Strategic Director of Knowledge at Equiniti
Equiniti is now within the strategy of migrating, consolidating and bringing all their information sources into the Databricks atmosphere and coaching and onboarding new customers and have numerous superior analytics and AI use instances within the pipeline to ship within the close to future.
This weblog was collectively authored by Tomasz Kurzydym (Senior Options Architect, Databricks) and James West (Strategic Director of Knowledge, Equiniti)