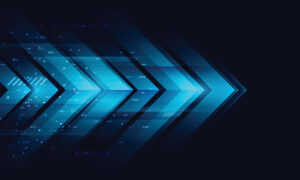
(DongIpix/Shutterstock
As prospects come to grips with the necessities of constructing and working generative AI functions, they’re discovering there’s one essential ingredient that makes all of it work: a vector database. That’s the primary issue driving adoption of this particular kind of database.
Whereas the sky-high hype round GenAI appears to be carrying off a bit, there may be nonetheless large curiosity within the nascent know-how.
As an illustration, a latest Boston Consulting Group survey discovered that IT leaders are projecting a 30% enhance in spending on GenAI and different types of machine studying within the coming 12 months, whereas a KPMG survey from March concluded that 97% of enterprise leaders plan to put money into GenAI over the following 12 months.
The momentum behind GenAI helps to energy curiosity in vector databases, too. Vector databases have been the preferred class of database for the previous 13 months, in accordance with the database trackers at DB-Engines.
The vector database pattern exhibits no signal of letting up. Gartner predicted a 12 months in the past that 30% of firms will use vector databases with foundational fashions by 2026, up from simply 2% in 2022.
The database trade is responding to this enhance in demand by ramping up manufacturing of vector capabilities, for each stand-alone vector databases in addition to multimodel databases that help vectors amongst different knowledge sorts.
Whereas there are tradeoffs between the 2 forms of vector databases, the multimodel path seems to be rising fairly quick. A brand new research from Forrester discovered that, by 2026, 75% of conventional databases, together with relational and NoSQL, will incorporate vector capabilities into their choices.
“Some organizations choose these databases as a result of they provide broader integration of each vector and non-vector knowledge, allow hybrid search, and leverage current database infrastructure,” writes lead Forrester Analyst Noel Yuhanna within the report, titled “Vector Databases Explode On The Scene. “Additionally, some multimodel databases at the moment are offering vector capabilities at no further price as a part of current licenses, additional enhancing their attraction to enterprises.
There are a number of elements that go right into a buyer’s resolution to make use of a multimodel database or a local vector database. If the appliance requires “distinctive efficiency and … low-latency entry to vector knowledge,” then a vector database could also be so as, in accordance with Forrester.
Variations in use instances may additionally lead a buyer to decide on one over one other. Conventional databases excel at powering functions, reporting, and enterprise intelligence, whereas native vector databases are designed for GenAI, search, and retrieval augmented era (RAG) functions.
A buyer with a lot of high-dimensional, complicated knowledge may additionally do higher with a local vector database. Forrester additionally notes that native vector databases additionally do higher with unstructured knowledge (textual content, paperwork, pictures, video, audio), indexing complicated knowledge, and integrating with machine studying instruments.
A standard database has a number of advantages of its personal, nevertheless. They’re designed to help transactions, which isn’t actually an idea in a local vector database, in accordance with Forrester. Additionally they typically have higher help for third-party tooling. If you wish to entry the info with SQL, a conventional database is your greatest wager; native vector databases are principally accessed through APIs. Multimodel databases fall someplace in between relating to advantages and disadvantages.
“Not like conventional databases, that are optimized for actual matches on structured knowledge, vector databases excel in performing superior similarity searches on complicated, high-dimensional knowledge,” Yuhanna and firm write within the report. “For instance, a vector database can rapidly discover all pictures in a database which are visually just like a given picture by evaluating their respective vectors inside seconds. The distinctive benefit of vector databases lies of their capability to help specialised vector indexes, facilitating fast processing of requests and delivering the excessive efficiency required for querying complicated knowledge.”
How native vector databases allow prospects to retailer, index, and search throughout vector embeddings is especially essential, in accordance with Forrester. Native vector databases function superior indexing and hashing strategies, “together with Okay-dimensional timber, hierarchical navigable small world (HNSW) graphs, locality-sensitive hashing (LSH), Fb AI similarity search (Faiss), and graph-based indexes,” the analysts write.
A number of the commonest use instances for vector databases embody RAG, picture similarity search, suggestion engine optimization, buyer expertise personalization, anomaly detection, search engine, and fraud detection. Forrester would suggest a local vector database or a multimodel database relying on the actual necessities of every prospects’ particular use case.
“Go for a local vector database should you require low-latency entry to giant volumes (tens of terabytes) of vector knowledge completely,” the corporate writes. “Nonetheless, in case your functions demand the combination of vector and non-vector knowledge, go along with a mulitmodel database with vector knowledge capabilities.”
Whereas scalability and efficiency come up repeatedly within the native-vs.-multimodel dialog, there are questions on simply how efficient any of the vector databases are on the excessive finish.
“Forrester’s conversations with purchasers recommend most vector databases haven’t but demonstrated high-end scalability and efficiency, significantly when dealing with billions of vectors or when coping with tons of of terabytes of information,” the corporate writes. “For optimum efficiency, be certain that vectors use optimized indexes and fine-tuned search algorithms and that they leverage GPUs and scale-out architectures the place relevant.”
Associated Objects:
Is the GenAI Bubble Lastly Popping?